Machine learning spots viral reservoirs
28 Nov 2018 by Evoluted New Media
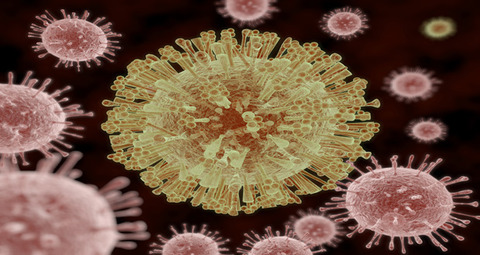
A machine learning algorithm has been developed at The University of Glasgow that can predict viral reservoirs in the animal kingdom.
Viruses circulate in animal and insect communities long before spreading to humans and causing severe disease. However, finding these natural virus hosts – which could help prevent the spread to humans – currently poses an enormous challenge for scientists. The new algorithm can use viral genome sequences to predict the likely natural host for a broad spectrum of RNA viruses, the viral group that most often jumps from animals to humans.
Dr Daniel Streicker, the senior author of the study from the MRC-University of Glasgow Centre for Virus Research, said: “Genome sequences are just about the first piece of information available when viruses emerge, but until now they have mostly been used to identify viruses and study their spread.
“Being able to use those genomes to predict the natural ecology of viruses means we can rapidly narrow the search for their animal reservoirs and vectors, which ultimately means earlier interventions that might prevent viruses from emerging all together or stop their early spread.”
The Researchers studied the genomes of over 500 viruses to train machine learning algorithms to match patterns embedded in the viral genomes to their animal origins. These models were able to accurately predict which animal reservoir host each virus came from, whether the virus required the bite of a blood-feeding vector and, if so, whether the vector is a tick, mosquito, midge, or sandfly.
Next, researchers applied the models to viruses for which the hosts and vectors are not yet known, such as Crimean Congo Hemorrhagic Fever, Zika and MERS. Model predicted hosts often confirmed the current best guesses in each field.
Dr Pete Gardner from Wellcome’s Infection & Immunobiology team said: “Healthy animals can carry viruses which can infect people causing disease outbreaks. Finding the animal species is often incredibly challenging, making it difficult to implement preventative measures such as vaccinating animals or preventing animal contact.
“This important study highlights the predictive power of combining machine learning and genetic data to rapidly and accurately identify where a disease has come from and how it is being transmitted. This new approach has the potential to rapidly accelerate future responses to viral outbreaks.”
The paper is published in Science and the code and data to replicate the analyses, add new data and improve the models is available.