Has Microsoft made an oncological smart bomb?
4 Nov 2016 by Evoluted New Media
In September, AstraZeneca and Microsoft made headlines for their pioneering ‘drag and drop’ computer model of cancer cell signalling. Here Jonathan Dry explains why this isn’t simply hyperbole and that we really could speed up cancer drug discovery by cutting out the ‘wet lab’
In September, AstraZeneca and Microsoft made headlines for their pioneering ‘drag and drop’ computer model of cancer cell signalling. Here Jonathan Dry explains why this isn’t simply hyperbole and that we really could speed up cancer drug discovery by cutting out the ‘wet lab’
Computer modelling of signalling pathways in cancer cells and simulation of experiments to test novel drug interventions look set to reduce the ‘wet lab’ work of drug discovery and may help boost our success rate in getting personalised cancer treatments to patients. At AstraZeneca, we are using a cloud-based modelling and simulation tool, called BioModel Analyzer (BMA), to gain new understanding of the millions of potential changes in cell signalling that make cancer cells multiply out of control.The original idea for this tool came from Jasmin Fisher, Senior Researcher at Microsoft Research and Associate Professor of Systems Biology at the University of Cambridge. Like us, she wanted to develop a biologist-friendly method of investigating the complexity of signalling pathways in a much more systematic way than has previously been possible. Computer modelling isn’t new, but many of the systems are mathematically heavy and difficult for biologists without a background in computer science to use. We needed a system that would ‘think’ like a biologist – not a computer scientist.
In effect, our computer screens become a blank canvas on which we first ‘drag and drop’ the essential components of the biological processes of cancer, such as cells, genes and proteins. Then we add the basic signalling pathways that can go wrong in cancer cells. It’s then over to the computer’s sophisticated algorithms to perform eye-watering numbers of calculations to fill in the gaps in the signalling pathways and predict what happens if different steps are blocked with drugs.
The pathways come to life, modelling logical relationships between many different proteins and simulating experiments that we previously had to do in the lab. We ‘test’ the likely effects of our drugs at different places on a pathway and identify the most promising places to intervene. In a signalling pathway that has become resistant to a cancer drug already in use, new opportunities are highlighted where we can add novel compounds with the potential to restore treatment sensitivity. Perhaps best of all, the information is presented in a visual, graphical way that is easy to understand.In effect, our computer screens become a blank canvas on which we first ‘drag and drop’ the essential components of the biological processes of cancer, such as cells, genes and proteins
Without computer modelling, drug discovery researchers have to rely on an incomplete understanding of the stages in cell signalling pathways and their own intuition to choose which steps to block. There may be 10s to 100s of different possible points to choose from in a single pathway. Even with the latest high throughput screening methods currently available it may take weeks of effort to identify promising candidates. With computer simulations, all that work can be done in minutes. Faced with an impossible number of different choices, scientists currently tend to choose their favourites to investigate and may therefore miss better options. The beauty of computerised modelling is that you remove the current limitation on the number of hypotheses you can investigate and the potential for human bias.
The pathways come to life, modelling logical relationships between many different proteins and simulating experiments that we previously had to do in the lab.
We chose cell signalling pathways in acute myeloid leukaemia (AML) to test our new ‘drag and drop’ approach to drug discovery. We needed to start with real data from real cells in order to understand the subtle differences in signalling between different AML cell lines. In the laboratory, we used a range of techniques, including DNA and RNA sequencing and protein arrays, to collect genomic, transcriptomic and proteomic data from AML cell lines, ready for processing.
Microsoft computer scientists used this information to develop highly sophisticated algorithms to model all the possible variations in a key signalling pathway in AML cells and the likely outcomes. After that, it was time to simulate experiments that would normally have to be done in the laboratory. The aim was to predict how drug combinations might be used to overcome resistance to individual drugs that target particular steps in cell signalling pathways in AML. We also wanted to know how this might vary between cell lines. Having done the computer simulations, we needed to compare our in silico data with results from in vitro tests in the laboratory.We were delighted to see an excellent match between the computer simulations and the laboratory findings. Indeed, I was surprised by the accuracy of the simulation. It told us which drug combination would make a cell sensitive to treatment and the protein changes that led to that cell becoming sensitive. Training computer models to map and interrogate signalling pathways requires a huge data set on the pathway of interest from multiple diverse cancer cells. It’s time consuming and not something that is done routinely in drug discovery laboratories. However, as our collaboration with Microsoft continues, specific oncology projects, for example, in breast cancer, are being prioritised for the simulation approach.
Being near neighbours in Cambridge has proved a big advantage for our collaboration with Microsoft. We contributed the big data sets and knowledge about the cancer signalling pathway in AML in order to fine tune the models, and Microsoft brought the software expertise. They captured the relationships between proteins in the pathway to build the model and run the simulation. We were then on hand to validate the results of simulations with our laboratory data. As well as having regular face-to-face and hands-on meetings, being able to address any problems by just ‘popping over’ was key to the rapid progress of the project. We could help the Microsoft team understand the signalling pathway data, while we were able to learn from them how to use BMA. As we move ahead, a member of my team will work alongside Microsoft’s experts one day a week, and learn from them directly, both in hands-on practice and to investigate the potential to re-use models already built by their community.
Using computer modelling to capture the great variability inherent in signalling pathways in cancer cells, and then simulating the effects of drug combinations at key points for individual patients, has the potential to push back the current boundaries of personalised medicine. At present, cancer treatments are targeted at single points in signalling pathways, based on genetic mutations in a patient’s tumour. But future personalised therapy is likely to be able to spread its effects to multiple points in a pathway identified by computer simulations and then confirmed in the laboratory. Ultimately, such simulations could even take account of interactions between the tumour and its broader environment, including host immunity and DNA damage response mechanisms.
We contributed the big data sets and knowledge about the cancer signalling pathway in AML in order to fine tune the models, and Microsoft brought the software expertise
We’re moving to a whole new level of understanding of the intricacies of drug targeting. We’re moving away from hitting a pathway just because we know it’s active. Instead, we’re identifying the best place to hit the pathway in order to get the best possible response for that patient. There is also the potential to improve our drug development success rate – by focusing our oncology research on the targets that matter most.
Author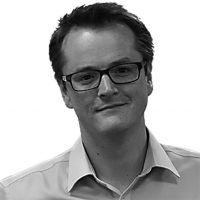