The surprising creativity of digital evolution
5 Oct 2015 by Evoluted New Media
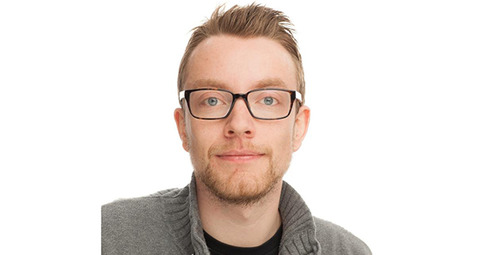
A creative machine may seem like somewhat of a paradox. We tend to think machines do strictly what they have been programmed to do. It turns out that machines can be quite creative, especially if they are simulating the most creative process known to man – evolution. Dr Kai Ellefsen and Dr Jeff Clune tell us more...
Evolution has produced an astounding diversity of functional, yet quirky creatures. They soar in the skies, explore the ocean depths, and roam forests, mountains, and plains. They also self-assemble from one tiny cell into many forms – baboons or blue whales – based on materials they (or their parents) collect from the environment. Were it not so familiar to us, the technology evolution has produced would seem like fantastical science fiction.
Because scientists and engineers wish to harness the power of nature to solve problems, they have replicated its basic principles inside computers. Evolutionary algorithms (EAs) simulate evolution, but allow the user to decide what makes something more fit, allowing the evolution of faster robots or cheaper microchips.
EAs have been applied to a wide variety of engineering problems, often producing solutions better than anything human engineers have come up with. They also allow us to conduct evolutionary experiments quickly, providing biological insights into how evolution works.
Talk to anyone who works with EAs on a daily basis and they will tell you that one of the great joys of working with “evolution in a bottle” is how creative it is. They’ll then grin and begin telling you stories of times that evolution surprised or even outwitted them. Here we wanted to share a few of those stories with you.
Elbow walking One of us ( Jeff Clune) was recently on a team of scientists that published a paper in Nature about how to make robots that, when damaged, learn how to change their behaviour to keep functioning despite the damage. Our main examples were six-legged robots that had to learn how to keep walking if motors in their legs broke. The key was using simulated evolution to learn a huge diversity of different ways for the robot to walk, so that, once damaged, it could test out very different strategies to find one that worked despite the damage. To evolve a diversity of gaits, we declared the fittest robots to be those that walked with their six feet touching the ground different percentages of the time. Naturally, we thought it impossible for evolution to solve the 0% case, but to our surprise, it did. Scratching our heads, we pulled up the video. It showed a robot that flipped over on its back and happily walked on its elbows with its feet in the air!
Playing dumb Charles Ofria (a former PhD advisor of Jeff Clune) once tried to test what happens with evolution if it is unable to improve on solutions. His organisms were small software programs that perform something similar to simple logic challenges. In his simulation, if a mutation produced a child program that gained the ability to perform logic operations (e.g. telling if two things were equal) its fitness would increase. Dr Ofria wanted to prevent that, so before releasing these digital organisms into the simulation he would give each child a test with logic problems to see if it gained a new ability. If it did, he deleted that organism. As expected, fitness stopped increasing. To Dr Ofria’s astonishment, however, after a while it started going up again. He looked into it and found that the organisms had evolved a knowledge of which questions were on the “test of new skills” and they played dumb, giving the wrong answers to those questions. Then, once they were cleared as not being any smarter, they were released into the virtual world, where they used their logic skills to better their station.
Thinking outside the box Researchers in Sussex, England, asked evolution to optimise the layout of a microchip to reliably distinguish two different sounds, but with thousands of times fewer components than is typically required. Evolution solved the problem, but to this day the researchers do not know exactly how it worked. Evolution did not even wire up key components known to be necessary to solve the task. The researchers suspect that evolution exploited magnetic flux (magnetic fields created by electrons flowing in the microchip) in ways we don’t fully understand to produce a solution unlike anything a microcircuit designer would dream of.
Scientists and engineers typically use evolution as an optimiser, meaning they try to harness and focus it by asking it to find the best solution possible for a specific problem. A new wave of researchers, however, is instead attempting to unleash the creative power of evolution and let it do what it does best: produce an unimaginable diversity of crazy, creative, quirky, yet fascinating and functional designs. These algorithms (e.g. Novelty Search or MAP-Elites) explicitly reward solutions that are different from others, rewarding them for being different just as mother nature rewards species that invade new, unexploited niches. While these algorithms often outperform traditional EAs, what’s even more interesting is the diversity of creative solutions they produce.
We believe this new approach opens an exciting new chapter in our field. As these new evolutionary algorithms increasingly unshackle evolution and let it run free, and given the power of modern computers to produce thousands of generations overnight, it will be fascinating to see what evolution comes up with next.
The authors:
Kai Olav Ellefsen recently completed his doctoral degree at the Norwegian University of Science and Technology, focusing on computer models of the evolution of learning abilities.
Jeff Clune is an assistant professor in the Computer Science Department at the University of Wyoming, where he directs the Evolving Artificial Intelligence lab (www.EvolvingAI.org).
Get in touch with us. We want to hear about your work, science passions and inspirations. phil.prime@laboratorynews.co.uk