Reproducibility comes as standard (part 1)
29 Jul 2019
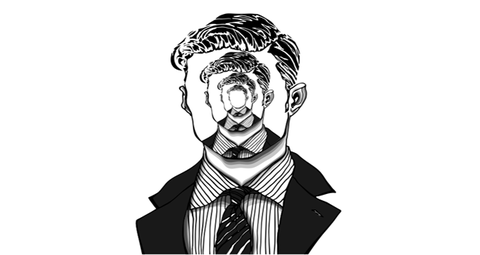
Science's reproducibility crisis is a big challenge and one that needs to be overcome quickly. In the first of a two-part feature, Gerhard Noelken from The Pistoia Alliance explains that the way data is recorded needs to change...
Unless the life science industry works as one to create ways to easily bring together data sets, not only will it miss out on the big data revolution, it’ll also fail to fix reproducibility issues
The amount of data we produce is growing exponentially; 2.5 quintillion bytes are created each day, and by 2020, it’s estimated 1.7MB of data will be created every second for every person on earth.
This rapid growth is acutely felt in life science R&D, where an abundance of real-world data is now available through channels such as wearable devices and health services data. In the laboratory as well, we see numerous small, high-quality data sets generated from individual experiments, but this data is locked in silos because of different data formats.
At best, time and resource is taken up trying to interpret different data formats, at worst, valuable data is ignored because it can’t be shared and understood
Traditionally, lab teams have not considered the long-term life of the experiment data they produce, but rather have created it for a specific project, with the sole goal, for example, of getting a therapy or drug to market as quickly as possible. As a result, metadata or ontologies are often skipped or incorrectly assigned when recording the method for an experiment, meaning the data can’t be reused.
These elements are essential when reproducing a method and experiment, as they provide vital context about the associations and relationships between items, so experiments can be validated in the future. A survey by Nature showed that 70 percent of efforts to reproduce experiments fail, exemplifying the extent of this challenge.
Researchers need access to consistent, high-quality data sets which can be combined to facilitate better analysis and enable them to draw conclusions and visualise trends. This is ever more important with the rise of artificial intelligence (AI), and deep and machine learning, which rely on quality data to be ‘trained’ with. When AI is used to make decisions about health, trusted data is paramount. Simply put, without data consistency, the vast amount of life science data collected in the laboratory might never be a player in the big data field.
MethodsDB to transform method capture
For life science R&D to improve data quality, the way data is recorded needs to change. The industry must move away from proprietary data formatting and adopt more consistent, even standardised data formats. Currently, there are no industry-wide standards that make data discoverable and sharable – whether it comes from regulators, partners, competitors, or internally. Data formats also vary widely between companies; some may be using ‘off the shelf’ tools, while others may have invested millions in building their own in-house systems. By making the shift towards standardisation, data will become interoperable, allowing machines and computational tools to understand and use it.
The Pistoia Alliance is working to address this challenge through its Methods Database (MethodsDB) project – beginning with analytical chemistry method capture. The project is in collaboration with the Allotrope Foundation, an international consortium of pharmaceutical, biopharmaceutical, and other scientific research-intensive industries and software companies. The project uses the Allotrope Data Framework (ADF) technology stack and forms a building block in the overall development of the Lab of the Future (LoTF), which aims to modernise lab environments through embracing technology, data, and automation.
The MethodsDB project will enable the digitisation of analytical method descriptions – making it easier to reproduce experiments on different instruments. Developing a universal solution that can store, search and retrieve a digital record of an experimental method would improve scientists’ ability to retain institutional knowledge, reduce time for method development, and improve the process for method execution. This will save scientists considerable amounts of time and cost, as well as improving accuracy of experiment outcomes, and their reproducibility.
The project was launched in recognition of the fact that method recapitulation is difficult and time consuming. In many cases, scientists still handwrite their methods, which can run to as many as eight pages long, and they may work with more than 30 instruments in a single lab, each with a different interface, resulting in an inordinate amount of time spent inputting variables. A digital instruction set that can be read by a machine reduces room for human error – it also enables the method and results to be linked together, creating an auditable trail.
Being able to reproduce experiments is essential to ensure drugs under investigation can move from the initial lab to a contract research organisation (CRO) and can be checked by regulatory bodies – it will also save time and resources spent on future method development. The initial proof of concept for the MethodsDB project is focused on High-Performance Liquid Chromatography and will be extended to other analytical methods in the future. It currently covers 30 parameters common across HPLC systems, using the Allotrope data format generating a single standardised way of recording the method.
Further steps towards the LoTF
The MethodsDB project is one of several that The Pistoia Alliance is undertaking in the drive to develop the LoTF and help life science organisations realise the value of their data – with another such project being the Unified Data Model (UDM). The UDM is a universal data format that was donated to The Pistoia Alliance by global analytics company, Elsevier; the project will create and publish an open and freely available data format for storage and exchange of experimental information about compound synthesis and biological testing. The UDM project is important because most companies have been using their own internal data formats, in which huge volumes of data have been generated and stored over the last 30 – 40 years, which will never see daylight.
Without standards like the UDM, there is a lack of consistency in data formats coming from different notebook systems and this makes it difficult to share experimental information. At best, time and resource is taken up trying to interpret different data formats, at worst, valuable data is ignored because it can’t be shared and understood. Importantly, it is collaboration across vendors and life science customers that has been the key to building a set of requirements that have driven the development of the UDM.
Unless the life science industry works as one to create ways to easily bring together data sets, it will never truly realise the potential of big data. To practically address the problem of limited and inconsistent data quality, common principles need to be adopted by all industry stake-holders. Such guidelines do exist, for instance, the FAIR data principles (Findable, Accessible, Interoperable, Reusable), but adoption must be further encouraged. The FAIR data principles were published in 2016 with the goal of emphasising machine-actionability (the idea that computational systems can access, interoperate and reuse data with minimal human intervention) as humans increasingly need computational support to manage the vast volume and complexity of data generated daily. The Pistoia Alliance is continuing to work with its members on how they can adopt FAIR.
Collaboration is critical
Ultimately, companies can no longer go it alone and must work together to develop standardised data formats in all areas – from method recapitulation to storage of real-world data – through projects such as MethodsDB and UDM, and initiatives such as FAIR. Each year, as the costs of R&D, regulatory demands and the need to prove economic value continue to increase life sciences companies are under pressure to deliver successful outcomes, and collaboration and data exchange will be fundamental to improving R&D success.
This project is a step in the right direction to correct these issues and to support the LoTF, with many big names in life science working together. To increase the effectiveness of life science R&D and continue to develop life-saving therapies for patients, there needs to be an industry-wide focus on collaboration.
The Pistoia Alliance is now looking to engage pharmaceutical companies, instrument vendors, and service organisations, to come forward and support phase two of the MethodsDB project.
Contact: MethodsDb@gmail.com
Author: Gerhard Noelken is a Consultant at The Pistoia Alliance - a global not-for-profit members’ organisation working to lower barriers to innovation in life science and healthcare