Reproducibility comes as standard (part 2)
5 Aug 2019
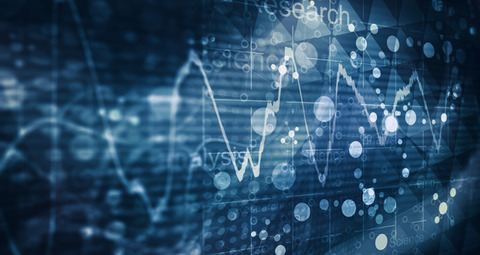
Science's reproducibility crisis is a big challenge and one that needs to be overcome quickly. In the second of a two-part feature on the subject, Rationally CEO Kristin Lindquist explains that the way we design studies needs to change...
In 2005, Stanford Professor John Ioannidis published his seminal paper Why Most Published Research Findings are False1 a compelling analysis of how current scientific practice results in findings that are more likely to be false than true. This replication crisis, as it is called, comes at a significant price - costing an estimate of $28 billion each year in preclinical biomedical research alone.2
Nature polled 1,500 researchers about causes and potential solutions to improve the reliability of published research. Problematic incentives (‘pressure to publish’) and various issues around methodology and reporting (‘selective reporting’, ‘low statistical power or poor analysis’, ‘poor experimental design’ and lack of ‘internal replication’) were identified as culprits.
Transparency, rigorous study design and good data analysis practices are the key to reproducibility regardless of discipline
Nearly 90% of respondents indicated ‘more robust experimental design’, ‘better statistics’, and ‘better mentorship’ as factors that would boost reproducibility. Relatedly, it was also found that causes of irreproducible preclinical research fell into four main categories: unreliable reagents and reference materials (36.1%), flaws in study design (27.6%), data analysis & reporting (25.5%), and laboratory protocols (10.8%).2
What is reproducibility?
But what is meant by reproducibility? First of all, it is important to remember that reproducibility is not the aim in itself so much as a proxy for truth: “If a finding can be reliably repeated, it is likely to be true, and if it cannot be, its truth is in question”.3 In order that a finding be reproduced, researchers must provide sufficient information about the original procedures such that they can be repeated – Goodman et al3 call this ‘methods reproducibility’.
Next is ‘results reproducibility’: when the procedure is followed, does it produce the same results? Even if the study replication produces roughly the same results, do independent researchers reach the same inferential conclusion as a result (‘inferential reproducibility’)?
Finally, does the finding persist from the lab into the real world? Or critically in preclinical research, what is the likelihood of translation from an animal model into human subjects (‘generalisability’)? These four faces of reproducibility should be top of mind, but especially in biomedical research due to the importance of not just evaluating, but operationalising the truth.
Preclinical reproducibility
While not the only field experiencing a replication crisis, preclinical biomedical research has experienced some of the highest profile replication failures. As one example, Amgen researchers reproduced a mere 6 of 53 (11%) foundational oncology studies4. Perhaps this is a result of the heightened reproducibility challenges of preclinical research, such as the “dizzying array of variables that can influence an experiment,” not all of which can reasonably be known or controlled5. Looked at through the lens of Ioannidis1, the preclinical phase may produce more unreproducible results because of the low ‘prior odds’ of early stage, exploratory research. When combined with selective reporting (i.e. being less inclined to report findings that disconfirm the hypothesis), these factors increase the density of false positives in the body of reported findings.
Confounding and low prior odds cannot necessarily be avoided, but they can be understood. If the context of the research makes false positive likely, then researchers have extra reason to scrutinize, rather than over-interpret, positive results (including in-study replication) and eschew selective reporting. Most importantly, care should be taken to reduce potential causes of false positive where possible and reasonable to do so. Transparency, rigorous study design and good data analysis practices are the key to reproducibility regardless of discipline.
Standards
To combat some of these issues for clinical trials, the research reporting guideline CONSORT was developed when medical research experts attempted to create a new scale of clinical trial quality. This group decided to focus on reporting guidelines, after concluding that key methodological elements underpinning such a quality assessment were poorly and inconsistently reported. Leading the way on reporting standards, the guideline has seen broad adoption and was designated a top health research milestones of the 20th century. Standards for other types of research have sprung up in recent years, such as ARRIVE for in-vivo animal research.
If seeking to improve research reproducibility, such community-developed and evidence-based standards are a good place to start. However, adherence still presents a challenge: even amongst CONSORT-endorsing journals, the reporting of key methodological items was still dismal. That is why we at Rationally built an app to help researchers adhere to the standards appropriate to their field of study. Our aim is that these methodological items be considered in the study design phase, before any data collection has taken place. Better to consider how to reduce study bias prospectively than be left to report avoidable suboptimal design decisions post-hoc.
Beyond standards, Rationally helps guide optimal experimental design decisions: how to approach randomisation and blinding, how to determine sample size for a properly powered study, choosing the proper experimental unit, and implementing formal experimental designs such as randomised block or crossover designs that help control random variables.
Reproducibility presents a costly challenge for preclinical research, but best-practices around experimental design and data analysis can help. Standards such as ARRIVE provide a framework for better design and reporting, and tools such as Rationally make standards abidance convenient.
References:
- PLOS Medicine, 2005, https://doi.org/10.1371/journal.pmed.0020124
- The Economics of Reproducibility in Preclinical Research. PLOS Biology 16(4): e1002626
- Goodman, Fanelli and Ioannidis 2016, Science Translational Medicine??01 Jun 2016:Vol. 8, Issue 341, pp. 341ps12 DOI: 10.1126/scitranslmed.aaf5027
- C. Glenn Begley and Lee Ellis Nature?volume?483,?pages?531–533?(29 March 2012)
- Samsa and Samsa 2019 Acad Med.?2019 Jan;94(1):47-52.
Author: Kristin Lindquist is founder & CEO of Rationally, a startup making reliable research design easier, more fundable and more visible.