(A)I must insist
10 Feb 2020
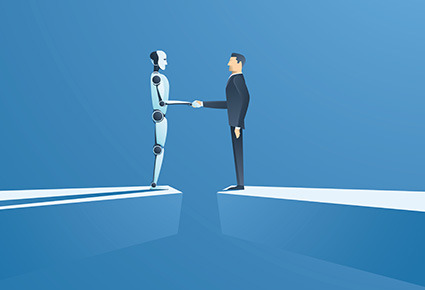
In a data-rich research landscape – only a mix of man and machine will give us hope of extracting knowledge from experimental data. Dr Paolo Mutti takes us behind the scenes at one of the World’s most complex experiments to show us why AI must be embraced by science
From autonomous cars to speech recognition, the use of Artificial Intelligence (AI) in science and technology is growing rapidly. Now, the implementation of AI technology in research can offer new opportunities for science facilities to supply cutting-edge science while accelerating the process of scientific discovery.
But despite these advantages, some scientific fields have not yet fully embraced AI techniques.
Scientific experiments in the next decade will be highly dependent on AI. From designing and manufacturing new technologies, to examining biological processes for health, AI will bring enhanced performance and productivity into the world of science. Already, AI has been used in multiple facets of astronomy, from modelling galaxy evolutions to optimising telescope set-up and introducing robotic control in land rovers, among many other applications. For large research facilities, AI may provide a solution for what is arising as a major challenge for the future: mass influx of data.
Scientific instruments are improving and data is being produced in higher volumes and complexity. In fact, the community has been faced with an explosion of data, both in the accumulation of data produced and the high-throughput of techniques and beamlines. Scientists must begin to tackle the challenge of analysing and fully exploiting this.
The need for speed
In November last year, experts from science facilities across the world gathered to discuss the future of AI in treating data at large-scale facilities. In a three-day workshop hosted at the European Photon and Neutron (EPN) campus and co-organised by the Institut Laue-Langevin (ILL), the European Synchrotron Radiation Facility (ESRF) and the Science and Technology Facilities Council (STFC), AI professionals and researchers came together to discuss how advanced computing power, new algorithms, and the exploitation of these technologies, can induce new discoveries in the field of neutron and X-ray science.
AI comes at a time where scientific output needs to be increasingly efficient and effective. Scientists are no longer able to trawl through all the data produced by their experiments. Further, traditional methods to analyse data have been selective. For example, analysis of diffraction data has always been focused on peaks in the dataset, but more recently, scientists have realised there is important information found in what was seen as background noise. In this aspect, deep learning methods are able to match and surpass human performance on image analysis and pattern recognition.
To fully analyse and exploit data, it is clear that facilities need to leverage computer-aided resources. Combining experiments with machine learning offers opportunities to support researchers in various stages of experimentation. New tools and methods provided by AI will not only allow scientists to fully explore complex datasets and draw benefit from them, but also at a faster pace and in higher volumes.
The AI challenge
Scientists at major experimental facilities, as well as the user community, still face a number of unique challenges before they can fully embrace AI methods into their experiments.
First and foremost, producing data using neutron and X-ray sources is expensive. In complex experiments, the costs of setting up and using the necessary equipment as well as preparing the sample material can be higher than in other fields. Fine-tuning intricate equipment can be time-intensive and take up precious beam time. This is clearly the two domains where AI-based approaches could have the biggest impact.
Nowadays, high volumes of experimental data are produced during the measurements but a complete set of complementary information about the experimental conditions (metadata) are often missing. Training neural networks requires fully-tagged data for pattern recognition. Therefore, the lack of tagging in incomplete data sets renders it redundant for such training, posing a further challenge to developing AI approaches for the technique in question.
Nonetheless, some of the long-standing obstacles to progressing machine-learning techniques for neutron and X-ray science are exactly the types of challenges that AI will solve. While costs of producing data can be expensive, thanks to the development in modelling and simulation techniques as well as in computational power, excellent data set for AI training purposes can be produced. In such a way AI will have the potential to enhance long-term productivity and maximise the use of resources. At the ILL, this is where we saw an opportunity to incorporate machine-learning techniques into our experimental program.
Deep learning for neutron science
At the ILL, deep learning techniques are being applied on our Small-Angle Neutron Scattering (SANS) instrument, D22. The ‘Swiss Army Knife’ of materials science, SANS can be used to probe hard and soft matter as well as crystalline and biological structures. Recently, ILL users ran SANS experiments to track molecules in type 2 diabetes,and identify the potential of silkworm proteins in hydrogels designed for in wound dressings. As such, the three SANS instruments of the ILL are in high demand.
SANS is a laborious neutron technique to set-up, due to the large number of possible instrument configurations. The quality of experimental results is highly influenced by instrument configuration, sample parameters, and the sample environment. Hence, much time is spent setting up the beamline. Early prediction of the material’s structure would help the selection of the best instrument configuration, increasing beam time productivity and the quality of data obtained. At the ILL, we saw this challenge to be best fit for the AI treatment.
Deep learning methods were used in a two-pronged approach – first, a neural network was trained to recognise the structure of the sample, and then a second one to predict the optimal instrument settings in order to obtain high quality scattering images. Once the neural network is trained, structures and settings are recognised in real time.
Such tools could also help interpret data from neutron scattering experiments. The raw data collected contains rich scientific information about the structure and dynamics – and therefore the properties – of materials under investigation.
The prototype of AI methods applied to SANS has already demonstrated good prediction abilities, and we now aim to incorporate more complex structures into the algorithm. By further developing such tools for the SANS technique, and other instruments, we hope to support scientists in obtaining higher quality data in a shorter time, facilitating data analysis and interpretation.
The next steps
It is clearly an exciting time for the community. The planets of scientific research are aligning –research infrastructures are being upgraded, big data produced is becoming more detailed, and data infrastructure and computer technologies are improving.
The next stage for AI is obtaining further appropriate data. Currently at the ILL, the neural networks in development have been trained only on simulated data – and the results verified on a few real data. Yet, the performance of neural networks should be validated with more real experimental data. While there is over 40 years of data at the facility, due to large volumes not being fully tagged, it cannot be used for neural network training purposes. A large volume of data isn’t enough by itself – scientific input, intuition, and human-built algorithms are required to start the process.
In the meantime, however, supporting collaboration between AI professionals and the research community is the way forward. Sharing data and coordinating activities will provide the diversity of scientific inputs and techniques to explore what problems might profit more from an AI approach. Such collaborations will pave the way for the future of AI in science, accelerating our abilities to maximise the use of experimental facilities and increasing the quality of scientific output.
Author:
Dr Paolo Mutti, Head of Scientific Computing at the Institut Laue-Langevin (ILL)