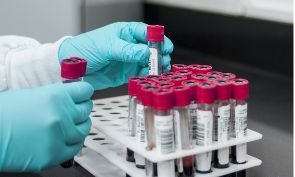
Scientific research takes time. But, as the Covid-19 pandemic highlighted, sometimes that’s a luxury scientists can’t afford, says Thibault Geoui. Experimentation and data guide us to the ‘right’ decision based on evidence. But progress can be slow, and in emergency situations like a disease outbreak, time is of the essence.
The pharmaceutical industry spends more than $200 billion annually on research and development, but investment in R&D is slowing. The mantra of ‘fail early, fail cheap’ feels apt, as researchers attempt to rapidly deliver high-quality candidates that reduce the risk of failure in clinical trials.
Consequently, there’s been a shift away from traditional in vitro and in vivo experiments towards more data-driven studies based on existing knowledge. Modern research solutions are now capable of integrating, analysing and comparing the huge volume of new scientific data generated daily. One such approach is in silico biology, an automated disease modelling methodology seen as a logical extension of controlled in vitro experimentation. It utilises computer algorithms to model pharmacologic and physiological processes, helping researchers understand disease biology and quickly identify potential therapies.
Disease modelling in Covid-19
The life sciences industry focused many resources towards finding potential treatments early on during the pandemic. An important first step is modelling the disease at a molecular level. Researchers at Elsevier combined prior knowledge and existing data with in silico biology to model the cytokine storm, a state of hyperinflammation observed in a small number of patients in the latter stages of a Covid-19 infection. Cytokines are a normal part of the body’s immune response to infection, but a sudden and uncontrolled release in large quantities can lead to multisystem organ failure and death. Once triggered, a cytokine storm is hard to stop.
Researchers used Elsevier’s Biology Knowledge Graph to identify the ‘Coronavirus infection’ and the proteins known to be regulated in response. (Knowledge graphs take data from published scientific literature, and enhance it with other information, such as experiment derived data from multiple sources.) From this, a conventional pathway of the disease was developed; this pathway can be thought of as a computer network made up of individual nodes, each of which can be altered and its effect on the network observed. In this way, the pathway becomes suitable for mathematical analysis and, importantly, machine readable. Researchers then have a time-saving tool to explain the dynamic behaviours of the disease and understand how it functions, without having to sift through data manually.
Researchers then have a time-saving tool to explain the dynamic behaviours of the disease and understand how it functions, without having to sift through data manually
A machine learning algorithm matched observed characteristics of the disease, as seen in experimental data, with those predicted by network regulatory logic. This allowed researchers to reproduce what they already knew about the disease and adjust the parameters to support observations, generating a model with few-to-no errors. After being validated against experimental data, researchers used the model to search for immune response behaviours most consistent with the known clinical pathology of Coronavirus infection, and examine how it responds to different therapeutic targets.
Fast-tracked therapies
Compounds from various drug databases and repositories populated with existing known molecules were then screened individually or in combination, due to their ability to redirect the disease state towards a healthy one. No drug, alone or in combination, is likely to meet all the criteria; rather the aim is to find something that enriches drug activity and drives the desired effect. By inputting numerous compounds into the in silico model, researchers examined the immune system’s response to various treatments, and demonstrated their mechanism of action as a potential therapy before moving to the lab. It also allowed them to show how existing drugs could be repurposed to treat the cytokine storm.
One drug showing promise was Ruxolitinib, a lupus treatment. Alone or in combination, the drug was shown to block certain signalling pathways and disable the regulatory machinery that triggers a cytokine storm. It’s now in Phase III trials for use in Acute Respiratory Distress Syndrome (ARDS), a common complication of Covid-19.
The latest addition to the R&D toolkit
Automated disease modelling tools like in silico biology are vital to the life sciences industry. They can accelerate R&D and support researchers attempting to answer global challenges, like finding therapies in crisis situations. Researchers are beginning to systematically use this cutting-edge method over other approaches because it incorporates vast amounts of untapped data generated in experiments and published in scientific papers, as well as data held in high-quality (and continuously updated) databases.
While in vitro and in vivo experiments take time, in silico biology can model the disease more rapidly and give researchers an understanding of the core regulatory network. This enables them to prioritise projects, identify ideal targets quickly, and forecast clinical side effects that could be missed during in vivo safety pharmaceutical studies, toxicology studies and clinical trials. And because the model can include an almost unlimited number of parameters, more ideas can be tested, results are more representative and have a greater statistical significance. The overall outcome is a faster, less expensive, and more accurate drug candidate selection process that leads to more meaningful predictions and has a positive impact on therapies.
Thibault Geoui is Senior Director, Discovery Biology and Predictive Risk Management at Elsevier elsevier.com/en-gb