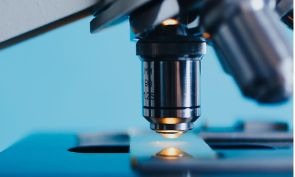
Improvements in technology, as well as data capture and analysis have increased the effectiveness of in silico testing. This has not only provided faster and cheaper trials but has significant benefits for patients too, explains Dr Gen Li.
Clinical trials are notoriously expensive, time-consuming and resource intensive. In addition, the obligation of participation from patients is considerable; one survey found almost half (49%) of trial participants reported that their daily routine was disrupted due to being involved in a clinical trial. The burden of being involved in clinical trials cannot be ignored; trial participants are subject to additional testing and observation combined with the uncertainty of being administered a new therapy that may not prove more efficacious than available standard-of-care treatments, or receiving a placebo drug. With this in mind, it is only right that this burden is kept to an absolute minimum when designing trials.
As the digitisation of R&D moves forward at pace, clinical development companies have the ability to harness data more effectively than ever before. By combining data with advances in technology as well as a willingness to update processes, the industry has an opportunity to use data to design patient-centric trials. With patient experience, an increasingly important metric in clinical trials, modelling and in silico analysis will become key in designing the clinical trials of the future. By enabling sponsors to simulate clinical trials, data is going to drive the transformation of clinical development to reduce patient burden and streamline processes.
Answering the ‘what ifs?’
Imagine a world in which the flaws, pitfalls and dead ends of a clinical trial were identified before a single patient was recruited. By knowing what the future holds, clinical development teams could design faster, more effective trials, involve fewer patients unnecessarily, and better recruit patients from diverse subpopulations. Using data to simulate trials provides this opportunity. By combining existing data – from patient records and historical clinical trials – with technologies such as AI and predictive modelling, clinical trials can be conducted in silico with reliable results.
By using the vast volumes of data held within existing electronic patient records (EPRs) there is an opportunity to update the clinical development process. There are sufficient data and tools available to facilitate in silico modelling before a wet trial ever comes to fruition. This makes the process much faster and reduces the number of patients that have to be put through a trial.
Moreover, it means that when protocols are written for a wet trial, they are based on data – meaning there will be fewer amendments during the trial process and the right patients are recruited first time.
With AI and machine learning, we have the power to dynamically design trials and really make the most of the existing data at every stage of the process. Not only does it save time and money by highlighting potential pitfalls and dead ends in the clinical development process ahead of time, but trial simulation also reduces patient burden substantially.
Phasing out the placebo
Thousands of clinical trials have been conducted in certain disease areas, each producing their own, comprehensive data set. However, despite the many thousands of trials completed, it is still common practice to include a placebo arm in randomised controlled clinical trials. But for well-studied disease indications, there is more than enough data available to accurately model how a control group will behave and avoid exposing patients to a placebo.
Synthetic control arms are an effective alternative to real-world control groups. By collating synthetic data from similar or identical trials with real-world patient data, comparator outcomes can be accurately modelled. Data can be used to identify potential side effect profiles, clinical outcomes, and inclusion and exclusion criteria before the trial begins. This reduces the need for patient involvement, saving time and overcoming the ethically questionable practice of giving placebos to patients who are in chronic and severe pain when there is enough historical placebo data from previous trials.
Eradicating the use of placebos and reducing patient burden should be an industry-wide goal
While synthetic control arms are hard to come by, as they must go through lengthy approvals processes via regulatory bodies, to construct digital twins is routinely feasible for most diseases. Using data collected from sources including EPRs, patient-generated data from fitness trackers or home medical equipment, disease registries, and historical and current clinical trial data, an ‘in silico patient’ can be developed. These digital twins can be employed to optimise development pathways, improving protocol design, and reduce patient burden by eliminating the need for a real person to go through the trial process.
An in silico toolkit
As technology continues to advance research and development, the opportunity to use data to predict outcomes, automate processes and optimise operations is undeniable. Integrated and automated data systems combined with analytical algorithms and statistical tools should be leveraged to accelerate the application of synthetic data. Working towards eradicating the use of placebos and reducing patient burden should be an industry-wide goal. With tools like digital twins, synthetic control arms and AI-guided machine learning, the next five to 10 years will be an exciting period of innovation for the clinical development industry, providing the opportunity to conduct clinical trials virtually before initiation. Making the real thing faster, lower cost and less of a burden on patients.
Dr Gen Li is President of Phesi