Like humans, LLMs are irrational but in different ways
9 Jun 2024
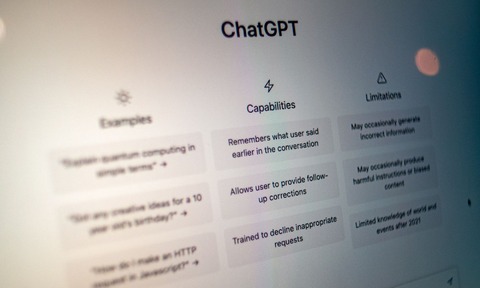
A study of several of the best-known large language models appears to confirm that, like humans, they can make irrational decisions but for quite different reasons.
The UCL computer science department test of seven LLMs published in Royal Society Open Science journal presented 12 cognitive psychology challenges to assess the presence of rational reasoning capacity.
This revealed widespread irrational responses and a considerable variation in the ability to provide correct answers to the particular tests.
First author of the study, Olivia Macmillan-Scott, said: “Based on the results of our study and other research on Large Language Models, it’s safe to say that these models do not ‘think’ like humans yet.
“That said, the model with the largest dataset, GPT-4, performed a lot better than other models, suggesting that they are improving rapidly.”
The authors said the data flagged the need to better understand how AIs ‘think’ before trusting them with decision-making tasks.
Issues highlighted included the tendency to provide different answers for the same reasoning test and failing to improve after receiving additional context.
For the research, the team defined a rational agent in terms of the ability to reason according to logic and probability.
The tests employed included mainstays of cognitive science including the Wason task and the Linda problem – both of which present significant challenges to humans attempting to solve these tasks. Recent studies resulted in just 14% of participants solving the Linda problem and 16% achieving the Wason task.
Yet the models also frequently exhibited irrationality in answers. These included providing different responses when asked the same question 10 times, as well as making simple mathematical and grammatical errors.
The Wason task revealed a very wide range in success, ranging from the 90% for GPT-4 to 0% for GPT-3.5 and Google Bard. Llama 2 70b, managed a little better than the latter two but answered correctly only 10% of the time and mistook the letter K for a vowel – a mistake few humans would make.
Said Macmillan-Scott "The model with the largest dataset, GPT-4, performed a lot better than other models, suggesting that they are improving rapidly. However, it is difficult to say how this particular model reasons because it is a closed system. I suspect there are other tools in use that you wouldn’t have found in its predecessor GPT-3.5.”
Some models also refused to answer innocent questions, citing supposed ethical grounds. And, whereas providing more contextual information has been shown to aid human respondents, the LLMs tested showed no improvement after such assistance.
Professor Mirco Musolesi, senior author of the study, admitted the capabilities of the tested models was “extremely surprising, especially for people who have been working with computers for decades".
“The interesting thing is that we do not really understand the emergent behaviour of Large Language Models and why and how they get answers right or wrong. We now have methods for fine-tuning these models, but then a question arises: if we try to fix these problems by teaching the models, do we also impose our own flaws?
He added that the behaviour of the LLMs allowed researchers to reflect on humans’ own reasoning and biases, and to consider whether fully rational machines were desirable:
“Do we want something that makes mistakes like we do, or do we want them to be perfect?”
The models tested were GPT-4, GPT-3.5, Google Bard, Claude 2, Llama 2 7b, Llama 2 13b and Llama 2 70b.
Pic: Emiliano Vittoriosi