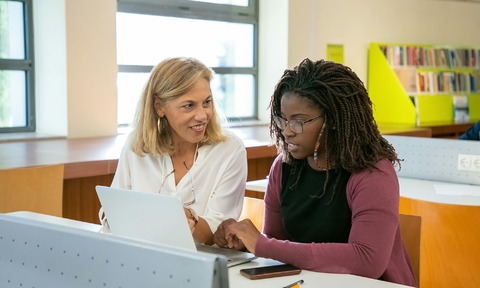
Our guest speaker for the annual Laboratory News session at CHEMUK was Finlay Morrison. His well-received insight, into the role of archive data transformation in unlocking the potential of AI-driven research, argues for a systematic approach to integrate legacy data into contemporary scientific workflows…
The evolution of laboratory research practices has significantly changed how research is performed. Where scientists used to record results and observations manually, we now have Electronic Lab Notebooks (ELNs) and Laboratory Information Management Systems (LIMS) that efficiently capture and organise large quantities of data.
Despite remarkable advances in laboratory technology, a substantial amount of valuable scientific information remains trapped in unstructured formats. Integrating this type of data into modern data management systems presents a formidable challenge. The inherent flexibility of unstructured data clashes with the rigid structures of current data management systems. Consequently, there is often a trade-off between capturing the narrative context of the work and recording only the essential data and metadata that fit into these systems.
Furthermore, many organisations are still grappling with fundamental questions about their data needs, adding another layer of complexity to an already intricate problem. As we look toward the future of laboratory research, unlocking the potential of unstructured data and developing effective integration strategies will be crucial for fostering a more efficient and data sources. innovative research environment.
One such example of data that presents difficulties integrating with modern datamanagement systems is the historical archives of handwritten lab notebooks. Archival data commonly does not adhere to the FAIR (Findable, Accessible, Interoperable and Reusable) data standard, which presents issues when working with it and thus remains under-utilised. Many of the final results of these experiments have found their way into scientific papers.
However, the published results typically only contain a small subset of the information gathered during experimentation, with much remaining hidden in researchers’ logbooks. The results that do not find their way into published papers are vital for telling the story of how the data was obtained and, therefore, could still be valuable for further research to reproduce the results or explore related research topics.
Nevertheless, this is a manageable problem. Recent advancements in the field of Machine Learning (ML) have paved the way for tools that can effectively organise information from unstructured data sources.
For example, object detection technology has made several significant strides in the past decade, particularly with the “You Only Look Once” (YOLO) network architecture. This architecture enables efficient extraction and classification of information from scanned pages by identifying regions of interest on the page. The regions can then be extracted, and the information can be further processed using techniques such as Optical Character Recognition (OCR) and Optical Chemical Structure Recognition (OCSR).
At Data Revival, we have worked with an archive containing over 4,000 handwritten lab notebooks, some of which had not seen the light of day for over three decades. We worked with 400 of these notebooks and identified over 17,000 handwritten molecular structures and 7,000 diagrams within them.
The potential of such enormous datasets cannot be overstated, so it is of paramount importance that we get systems in place which properly incorporate this information into the research flow for the lab of the future. By doing so, we can ensure that the valuable knowledge contained within this data is not only preserved but also actively utilised.
OCR and OCSR can process the previously identified text and chemical structures. OCR determines the text in an image by predicting a sequence of tokens, each representing one or more characters. The types of text found in chemical data are atypical compared to the general use of text, particularly the use of long chemical names. For this reason, OCR models must be trained to work well with chemical-related text. OCSR works by predicting a line-notation chemical sequence, such as SMILES or InChI, from an image of a chemical molecule. Since both OCR and OCSR predict something representable by text from an image, many techniques and neural architectures are transferable between the two tasks.
Despite remarkable advances in laboratory technology, a substantial amount of valuable scientific information remains trapped in unstructured formats
Although only OCR and OCSR have been explained here, one can imagine an extensive range of dedicated models to extract the specific types of information that may be relevant to a given archive. For example, you could have a model dedicated to reading information from chromatography plots or extracting information from graphs.
After information, such as written text, chemical formulae, or graphs, has been extracted and transformed into a computerunderstandable format. Large Language Models (LLMs) have demonstrated potential in organising this information into formats that integrate smoothly with contemporary data management tools. Additionally, we have seen that these models can dr aw in a large amount of context and their tr aining to spot and correct minor mistakes in the previously described OCR technique.
The techniques explained so far have been aimed at incorporating historical data into contemporary data management systems. However, these methods are not exclusive to archive data. They could allow a more flexible method of data logging in the lab of the future, enabling research workflows unencumbered by the rigid framework prescribed by tools like ELNs, whose strict layout may lead to valuable data being improperly recorded if it does not fit into their structure.
Additionally, automated ontological development will enable researchers to efficiently use data across the many domains they explore, facilitating cross-disciplinary insights and accelerating the speed at which new products or processes can be developed.
Looking ahead, the potential applications of these datasets are immeasurable. They can fuel data-mining pipelines that identify patterns and opportunities for further experimentation. They can serve as the foundation for training research-focused language models.
At Data Revival, we envisage a future where AI-based systems are key partners in discovery. Imagine intelligent systems that assist scientists in planning and executing experiments, drawing from a vast corpus of past experiments.
Imagine multimodal models capable of processing data in various formats, such as text, images, or sensor readings, to gather insights on a scale beyond human capability. It’s a challenging task, but one that we believe to be instrumental in the future of scientific research.
Finlay Morrison is a data scientist at Data Revival and an electrical engineering student at the University of Southampton