LLMs’ neuroscience predictions leave the experts trailing
1 Dec 2024
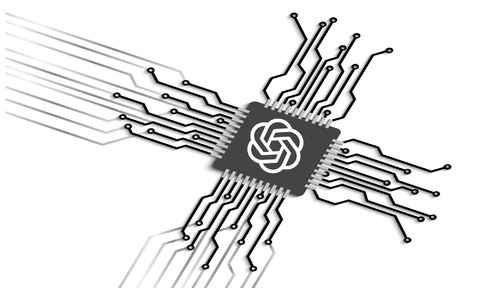
They’ve long since proved their effectiveness in information retrieval. Now large language models have outstripped humans at predicting science study outcomes.
A UCL team led by psychology and language sciences department professor Dr Ken Luo, challenged LLMs to predict the results of neuroscience study proposals, in competition with leading academics in the field.
While the neuroscientists managed a creditable 63% average accuracy rate, rising to 66% in the case of domain experts, their AI rivals did far better with a score averaging 81% accuracy.
Luo commented: “Since the advent of generative AI like ChatGPT, much research has focused on LLMs' question-answering capabilities, showcasing their remarkable skill in summarising knowledge from extensive training data. However, rather than emphasising their backward-looking ability to retrieve past information, we explored whether LLMs could synthesise knowledge to predict future outcomes.”
While scientific progress often relied on trial and error, the necessary experimentation demanded time and resources, he added.
“Even the most skilled researchers may overlook critical insights from the literature. Our work investigates whether LLMs can identify patterns across vast scientific texts and forecast outcomes of experiments.”
Senior author Professor Bradley Love of UCL psychology and language sciences said the findings increased the likelihood of researchers employing AI to design the most effective experiments. He continued saying that, while the study focused on neuroscience, the approach might apply across the science spectrum.
But, cautioned Love, the research published in Nature Human Behaviour might raise implications too regarding the quality of scientific output.
“What is remarkable is how well LLMs can predict the neuroscience literature. This success suggests that a great deal of science is not truly novel, but conforms to existing patterns of results in the literature. We wonder whether scientists are being sufficiently innovative and exploratory,” said Love.
Led by UCL the international research team developed its BrainBench tool to evaluate LLM predictive performance. This comprised pairs of neuroscience study abstracts: one genuine and outlining the research background, methods and results; the other with the same first two elements but with results modified by domain experts to yield “plausible but incorrect” outcomes.
A total of 15 general purpose LLMs and 171 human neuroscience experts were asked to discern which one of each pair contained the correct results.
Afterwards, the scientists adapted a version of open-source LLM, Mistral, honing it on neuroscience literature. The adapted LLM, titled BrainGPT, exceeded the 83% score by the general purpose Mistral LLM, achieving 86% accuracy.
Funding came from the Economic and Social Research Council (ESRC), Microsoft, and a Royal Society Wolfson Fellowship. In addition to UCL, research team members came from Cambridge and Oxford universities, the Max Planck Institute for Neurobiology of Behaviour in Germany, Turkey’s Bilkent University as well as other institutions in the UK, US, Switzerland, Russia, Germany, Belgium, Denmark, Canada, Spain and Australia.
Pic: Shutterstock (Pixathon)