Machine learning plus vital signs provides objective patient pain levels
18 Mar 2021
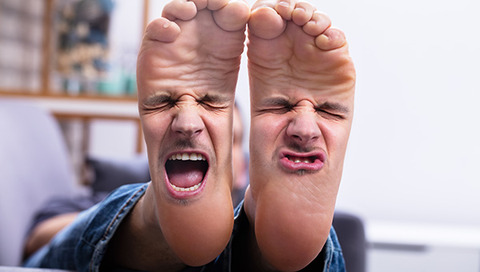
Describing pain from zero to ten is currently as good as it gets on the hospital floor when it comes to understanding a patient's pain levels and is very subjective. One person's two is another person's five, and try asking a two year old that same question. But now, a research team has found it’s possible to understand a patient’s pain level objectively by examining data from vital signs.
In a new study, led by Northwestern University faculty and alumni, has developed and applied artificial intelligence (AI), or machine-learning, algorithms to physiological data — including respiratory rate, blood pressure, heart rate, body temperature and oxygen levels — from patients with chronic pain from sickle cell disease. Not only did the researchers’ approach outperform baseline models to estimate subjective pain levels, it also detected changes in pain and atypical pain fluctuations.
The study is the first paper to demonstrate that machine learning can be used to find clues to pain hidden within data from patients’ vital signs.
Currently, patients must assess their pain based on a scale of zero to 10. This can be a difficult task because many people experience pain differently, and young children and unconscious patients cannot rate their pain at all. The researchers believe these subjective assessments of pain could be supplemented with a more objective, less invasive, data-driven approach to help physicians more precisely treat pain.
“Pain is subjective, so it’s tricky to assess when trying to treat patients,” said Northwestern’s Daniel Abrams, senior author of the study. “Doctors don’t want to undermedicate patients and not provide enough pain relief. But they also don’t want to overmedicate their patients because there is a risk of side effects and addiction.”
“Our study shows objective physiological data that is routinely collected at hospitals contains clues about a patient’s subjective pain,” said Mark Panaggio, the study’s first author. “We hope our work will inspire continued development of models to infer and ultimately forecast pain and that these models will allow clinicians to provide more timely and targeted treatments.”
Abrams is an associate professor of engineering sciences and applied mathematics at Northwestern’s McCormick School of Engineering. Panaggio is a former PhD candidate from Abrams’ lab; he is now an applied mathematician at the Johns Hopkins University Applied Physics Laboratory.
Study design sampled more than one hundred hospitalisations
To conduct the study, the researchers used data from patients with sickle cell disease who were hospitalised at Duke Medical Center due to debilitating pain. The sample included data from 105 hospitalisations of 46 distinct patients. When health care workers routinely collected the patients’ vital signs, those patients also rated their subjective pain levels.
To simplify the task, the researchers divided pain levels into three categories: low, moderate and high. After using machine-learning strategies to mine the data, the researchers compared their model’s assessment of pain to the patients’ subjective reports.
“Our model’s inferences did reflect the subjective pain reports,” Abrams said. “It was even more accurate at detecting whether a patient was above or below their normal level of pain.”
Although hospital data can be difficult to acquire due to confidentiality issues, Abrams, Panaggio and their collaborators are in the process of obtaining a much bigger dataset with pain reports from hundreds of thousands of patients with pain due to sickle cell disease and other causes, including post-operative pain and pain from unknown sources.
The researchers next aim to use their model to try to predict how pain relievers might affect pain and to forecast when patients with chronic pain might experience an excruciating flare-up, which is currently nearly impossible to predict.
“A large fraction of people with chronic pain go to the emergency room with pain crisis events, in which pain becomes unmanageable with prescription medications,” Abrams said. “Right now, no one knows what causes these events. If we could predict those events, we could save patients a lot of pain and money.”
The study, 'Can subjective pain be inferred from objective physiological data? Evidence from patients with sickle cells disease,' was published in the journal PLOS Computational Biology