Estimate your probability of success with Bayesian statistics
18 Jan 2022
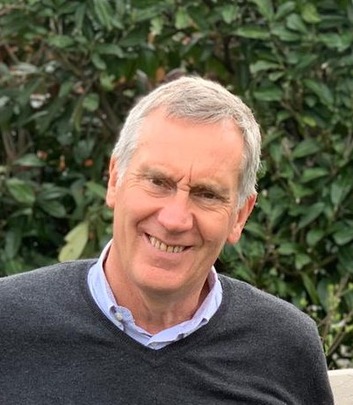
Don’t settle for approximations, make smart, risk-based economic decisions. Bruno Boulanger explains how the application of Bayesian statistics can assist with decision making when data samples are small but pre-existing information is available
By estimating the probability of future success, Bayesian statistics can help companies make smart decisions across many key areas.
Mathematical methods have long been used to assist with decision making in clinical research, with researchers often depending on the p-value, or observed significance level, to test statistical significance. However, this methodology relies on having large data sample sizes; whereas very often that isn’t the case, which makes it impossible to produce a reliable inference.
By applying a Bayesian approach, statisticians can make sense of small data samples by borrowing strength from across similar, but not identical, bits of information. In Bayesian statistics, previous and related information is relevant. For example, researchers might look at information from previous trials, scientific literature, or real-world data as part of an ongoing stream of data to achieve direct probability statements about unknown information, rather than settling for approximations.
Effective decision making is crucial during the clinical trial process, for example. Bayesian statistics address the probability inference for questions around the likelihood that a new drug is safe and effective, or that it will be successful, by leveraging pre-existing information about a molecule or therapy. By leveraging prior knowledge, Bayesian statistics allow researchers to reduce the number and size of clinical trials by injecting flexibility into the way the trial is designed. This both ensures projections aren’t overly optimistic and accounts for the probabilities of unknown issues occurring. Having fewer and smaller clinical trials is not only financially beneficial but also potentially more ethical, particularly when conducting studies into treatments for rare or paediatric diseases, where patients are often extremely vulnerable.
As pressure to improve the framework for making decisions has intensified, many biopharmaceutical companies are now seeking statistical experts specialising in Bayesian methodology to help them better weigh and address complex problems.
Bayesian statistics can be, and is being, applied across multiple parts of the product lifecycle. By estimating the probability of future success, Bayesian statistics can help companies make smart decisions across many key areas. It can help companies make risk-based economic decisions, such as whether or not to build a manufacturing line for a drug in development or for portfolio management.
Author: Bruno Boulanger is Global Head Statistics and Data Science at PharmaLex